wuvin
Always take risks!
Toggle navigation
wuvin
主页
实验室的搬砖生活
机器学习
公开的学术内容
公开的其他内容
About Me
归档
标签
友情链接
ZYQN
ihopenot
enigma_aw
hzwer
杨宗翰
NeurIPS 2022 NeRF 相关
2022-11-25 13:34:40
207
0
0
wuvin
翻译自 https://markboss.me/post/nerf_at_neurips22/ * 这边开始偷懒,只会点开部分我感兴趣的文章。不感兴趣的就只是翻译一下。 ### Neural Transmitted Radiance Fields * 针对性优化了折射和反射 * 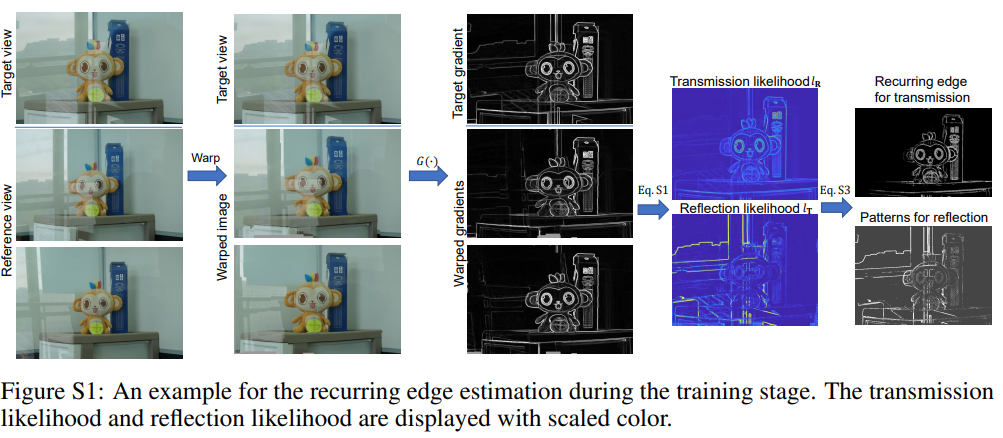 * 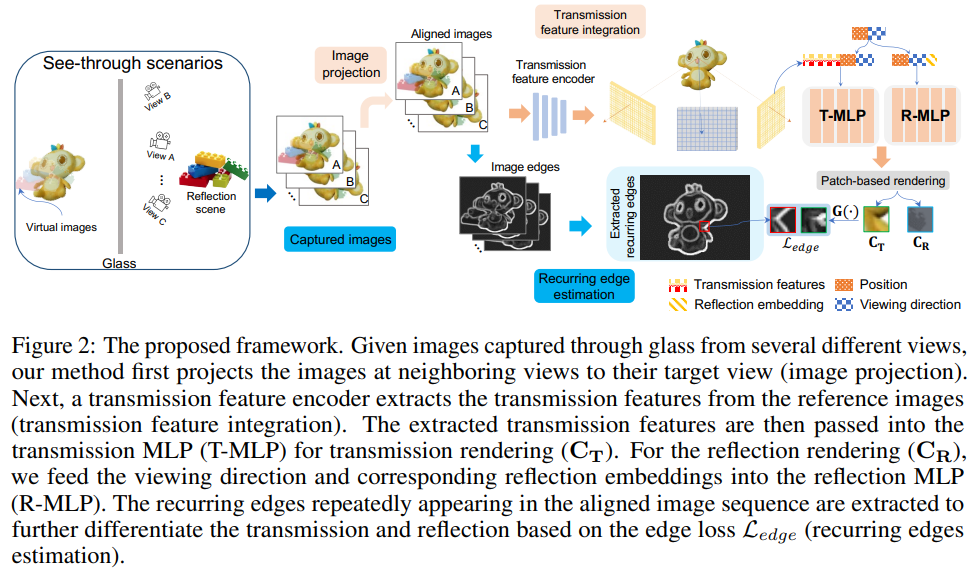 *  * 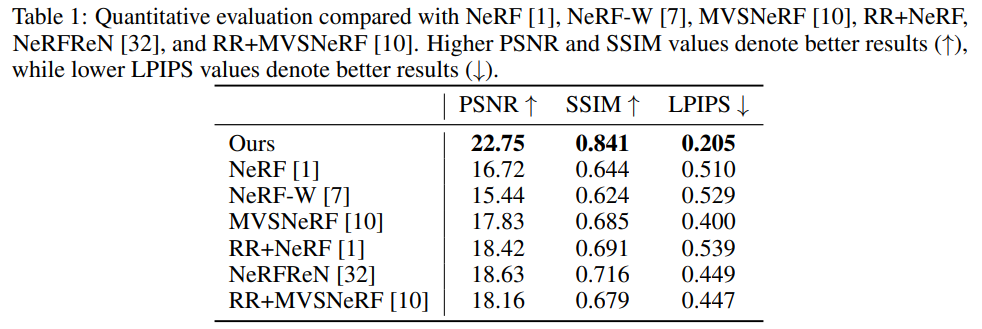 ### Polynomial Neural Fields for Subband Decomposition and Manipulation * 在隐式表征中引入了多项式,提升了可解释性,性能提升不明显 * 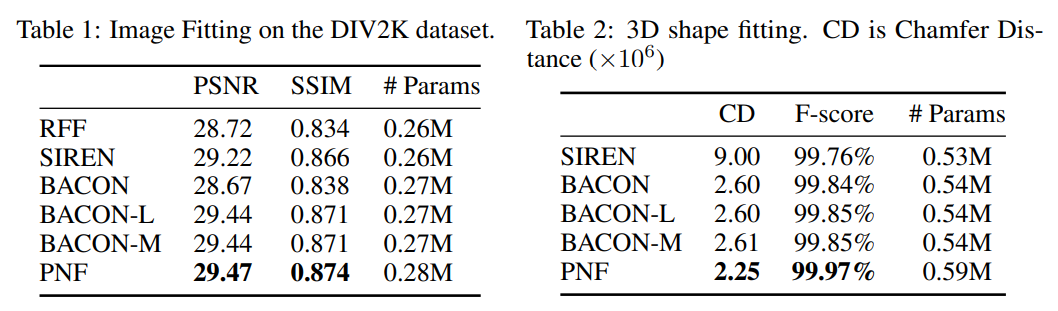 * 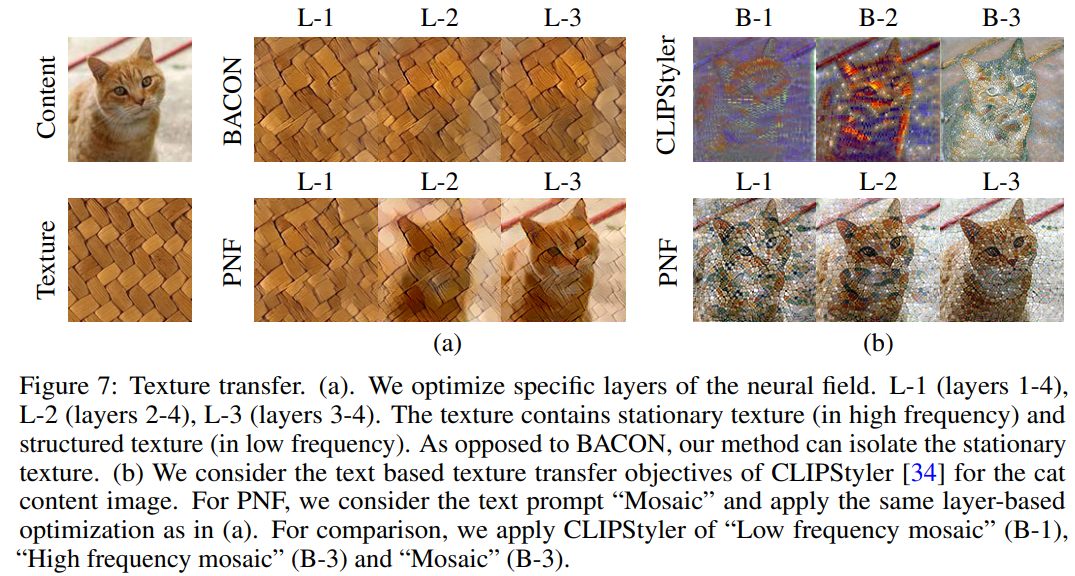 ### Implicit Neural Representations with Levels-of-Experts * 对空间依赖的MLP的分级细化,没做三维的 * 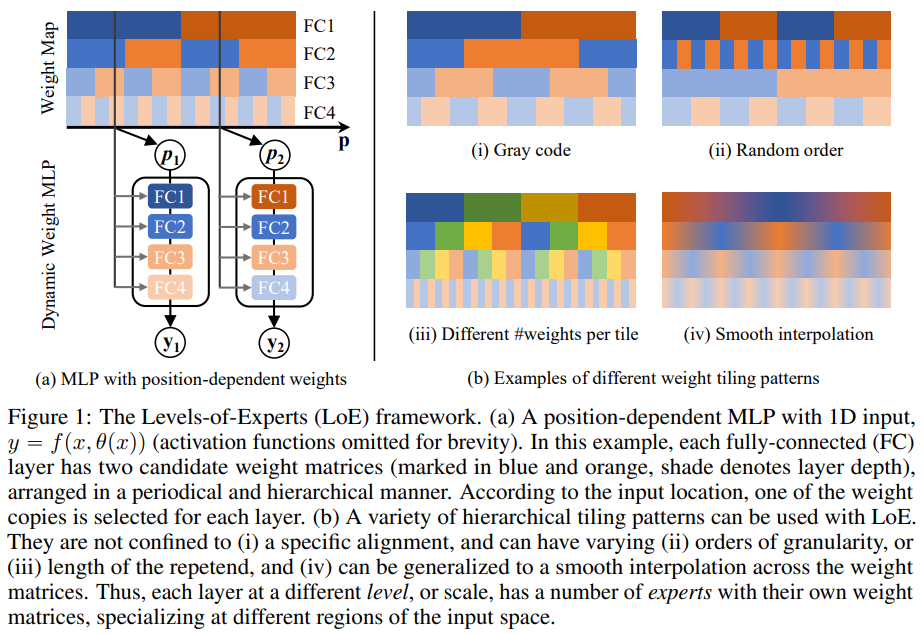 ### Generalised Implicit Neural Representations * 本文大胆地超越了欧几里得坐标系的范畴,提出将连续的高维信号观察为离散图,并对每个节点进行光谱嵌入,以建立神经场的输入。 *  ### HF-NeuS: Improved Surface Reconstruction Using High-Frequency Details * 对形状的高低频分离,用的SDF,或许在去噪三维生成上会一定启发 * 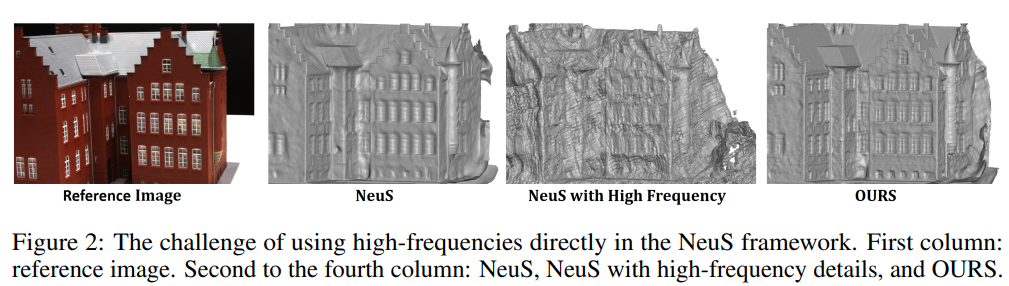 * 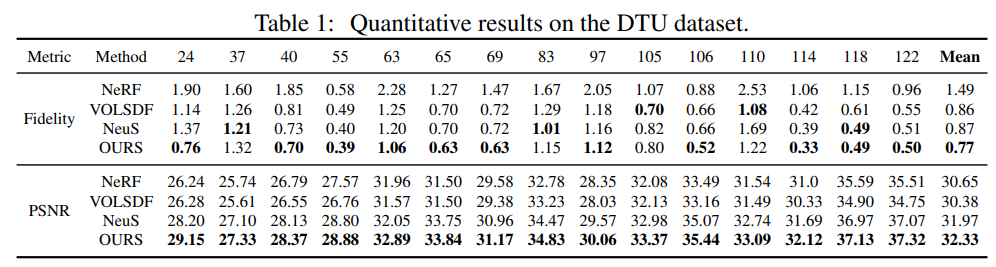 ### Geo-Neus: Geometry-Consistent Neural Implicit Surfaces Learning for Multi-view Reconstruction * 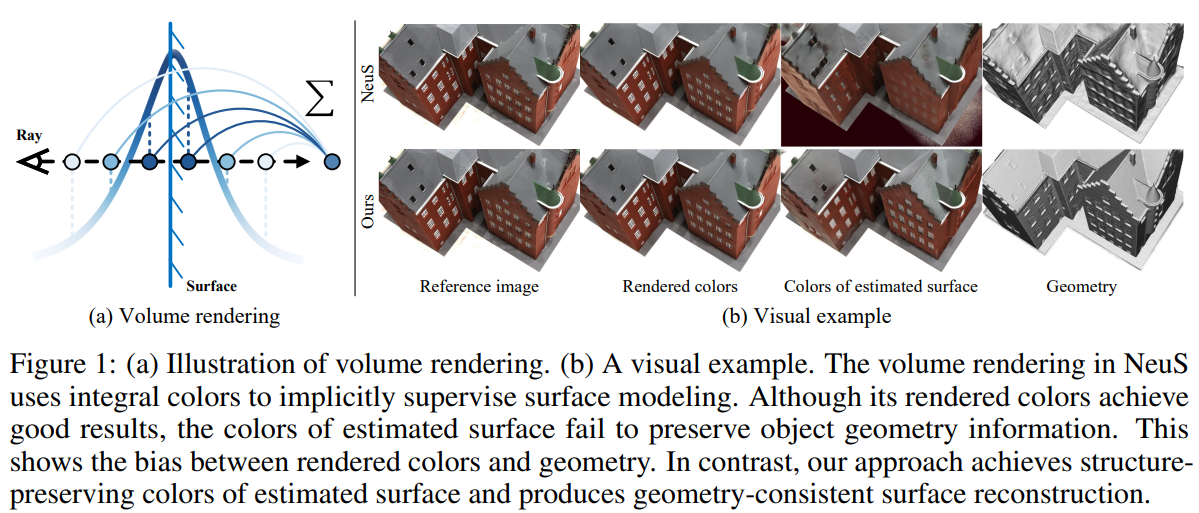 * Geo-Neus引入了明确的多视图几何约束,以生成几何上一致的表面重建。这些损失包括来自稀疏运动结构(SFM)点云的有符号距离函数(SDF)和光度测量一致性的损失。 * 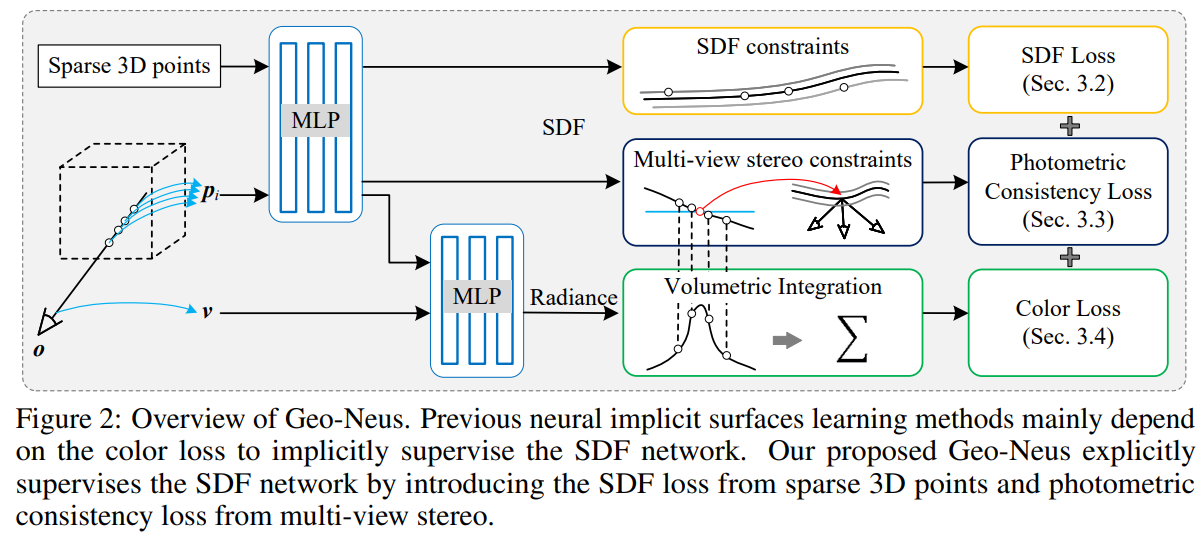 * 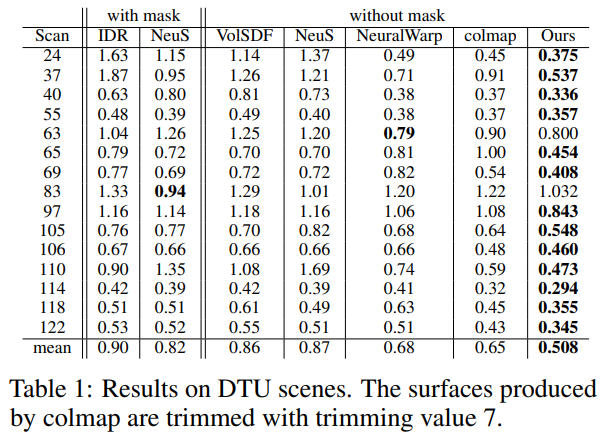 ### HSDF: Hybrid Sign and Distance Field for Modeling Surfaces with Arbitrary Topologies * SDF 只能建模封闭表面,本文采用混合符号函数处理复杂几何 * 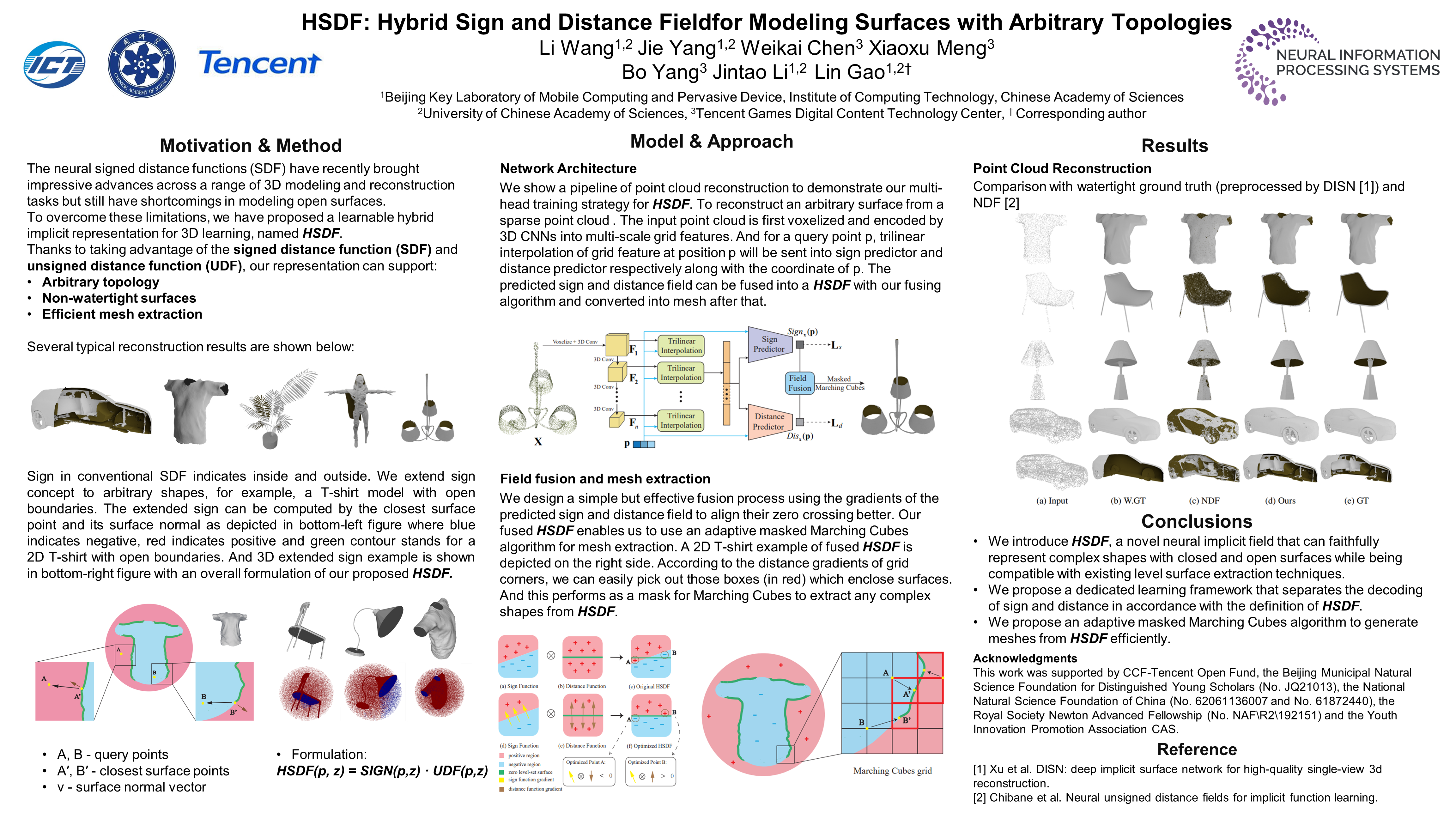 ### INRAS * INRAS使用神经场在任意位置存储高保真时域脉冲响应。这允许对一个场景的空间声学进行有效的建模。 * 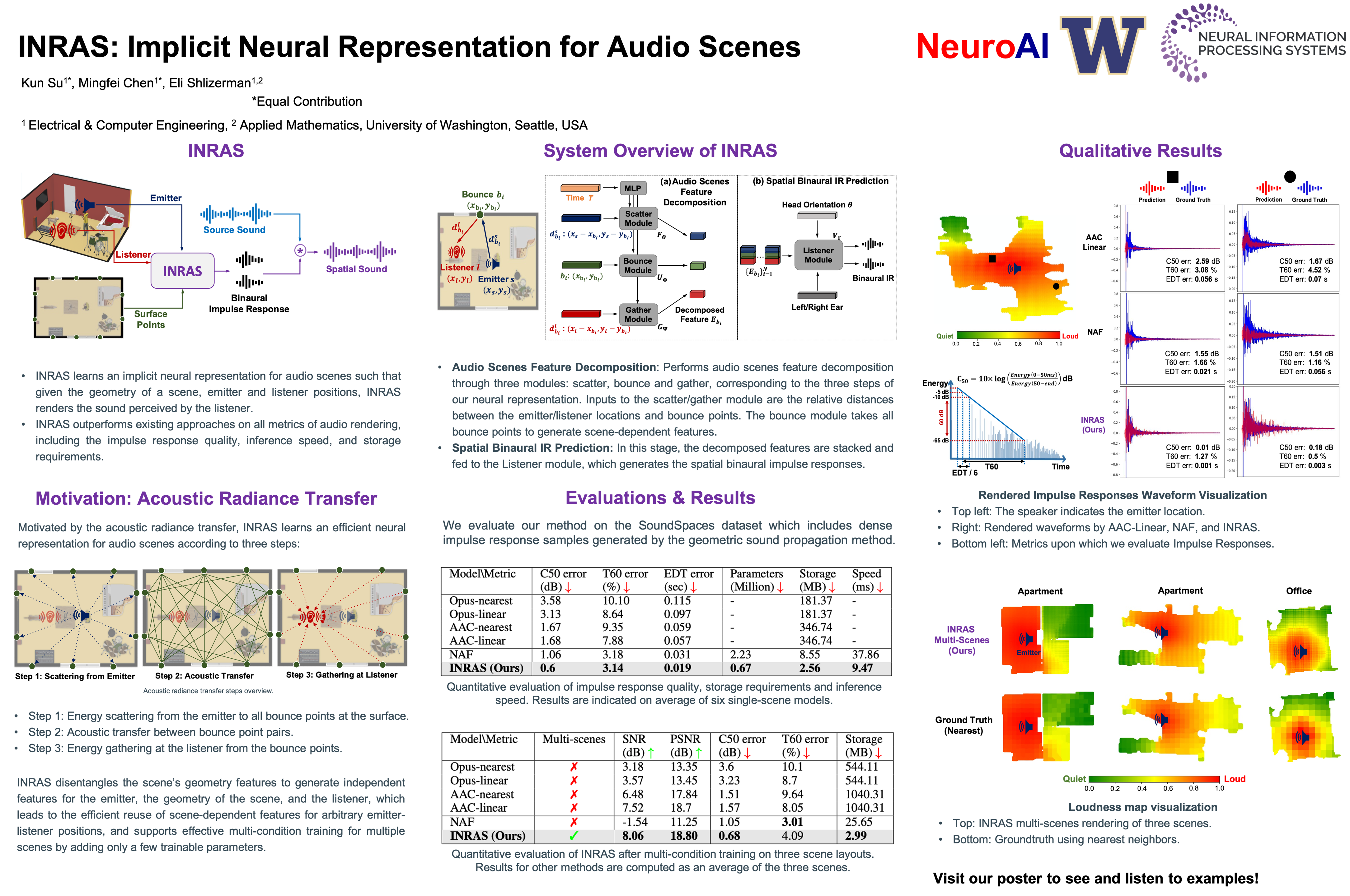 ### NAF * NAF遵循与INRAS类似的方法,在空间变化的场景中学习脉冲响应函数。 *  *  * 竟然还有跨模态的学习 *  ### Coordinates Are NOT Lonely - Codebook Prior Helps Implicit Neural 3D representations * 在CoCo-INR中,使用了两个注意力模块。一个用于从先验编码簿中提取有用的信息,另一个用于将这些条目编码到场景中。有了这个先验,该方法就能在稀疏的图像上工作。 *  *  ### NeuForm * 在稀疏的图像集合中,一些区域往往很少被观察到。为了规避这个问题,NeuForm在观察较少的区域依靠可概括的特定类别表示。在观察良好的区域,该方法使用NeRF的精确过拟合。 ### MonoSDF * MonoSDF整合了最近的单眼深度和正常预测网络作为先验因素。鉴于这些额外的先验,作者展示了在MLP神经场或基于体素的网格方法下的性能改进。 *  *  *  ### VoxGRAF: Fast 3D-Aware Image Synthesis with Sparse Voxel Grids * VoxGRAF结合了最近的方法,利用基于体素的结构和三维卷积来加快NeRFs的速度,从而在一次向前传递中产生新的物体。然后,这些场景可以从任何视点进行渲染。 *  *  *  *  *  * 结合了体素和GAN来实现,先从noise 生成带颜色(3 channel)和密度(1 channel )的三维体素,然后可微渲染得到二维图像,并交给discriminator。 * **这应该是看到和我们想法最接近的了**。 ### Neural Shape Deformation Priors * 在数据集上学习非刚性形变的先验。 *  ### NeMF * NeMF学习了人类和四足动物运动的先验,并在神经运动场中生成性地使用它。作者展示了用于运动插值使用案例。 *  ### DeVRF * DeVRF提出使用三维空间体素网格与四维变形场一起,以高效的方式对动态场景进行建模。 *  *  ### FNeVR * FNeVR通过将二维运动扭曲与神经渲染相结合,实现了用NeRFs为人脸制作动画。 *  ### NDR: Neural Surface Reconstruction of Dynamic Scenes with Monocular RGB-D Camera * NDR使用单眼RGB-D相机联合优化了动态场景的表面和变形。他们提出了一个可逆变形网络,以提供帧间的周期一致性。由于拓扑结构在动态场景中可能发生变化,他们还采用了一种策略来产生拓扑结构的变体。 *  *  ### D2NeRF * D2NeRF从单眼视频中学习静态和动态物体的解耦。在这里,两个网络被分别训练,它们分别处理各自的领域。 *  ### NeuPhysics - Editable Neural Geometry and Physics from Monocular Videos * NeuPhysics允许通过编辑物理参数来编辑一个动态场景。这种编辑是在一个六面体网格上进行的,与底层神经场进行双向转换。物理模拟是在网格上以不同的方式进行的,并且可以传播到神经场。 *  ### CageNeRF: Cage-based Neural Radiance Field for Generalized 3D Deformation and Animation * 这个和 eccv 的那个重了吧 * CageNeRF使用对象周围的低聚物笼子来创建一个简单的变形目标。然后在手动编辑中对笼子进行变形是很简单的。然后,该变形可以用来对先前训练的NeRF进行扭曲。 *  ### Signal Processing for Implicit Neural Representations * ** 很新奇的想法** * 把一个普通NeRF MLP作为输入,对于每一个计算都对应了额外的网络结构,就像构造计算图一样。但这么做的意义在哪呢?无非就是把 $y = f(x)$ 中的 $y$ 用 $f(x)$ 替代。 * INSP-Net通过引入微分算子框架将信号处理引入神经场,它可以直接在场上工作而不需要离散化。作者甚至提出了一个运行在神经表示上的CovnNet。 *  *  ### Decomposing NeRF for Editing via Feature Field Distillation * 看过的,CLIP-NeRF 相关 * 本文使用现有的二维特征提取器,如CLIP-LSeg,以提供额外的监督来检测三维体积中的语义。用户可以根据文本、图像补丁或直接像素选择进行查询,以允许语义编辑。 *  ### Compressible-composable NeRF via Rank-residual Decomposition * 压缩 NeRF 大小 * CCNeRF使用张量等级分解,以高度有效和可压缩的方式表达神经场。由于表示是明确的,所学的模型也是可组合的。 *  *  *  ### Rotation-Equivariant Conditional Spherical Neural Fields for Learning a Natural Illumination Prior * RENI使用SIREN和矢量神经元来学习关于自然HDR照明的神经先验。他们扩展了矢量神经元以处理球形图像的旋转等值。 *  *  ### Neural Reflectance Field from Shading and Shadow under a Fixed Viewpoint * 本文利用了一个固定的视角和一个移动的光源。这样一来,几何图形和BRDF就必须根据阴影线索来恢复。由于神经场的作用,即使从固定视角也能恢复三维场景。 *  *  ### SAMURAI: Shape And Material from Unconstrained Real-world Arbitrary Image collections * 在SAMURAI中,该模型可以将粗略摆放的图像集合分解为形状、BRDF和光照。在训练过程中,相机也会通过相机复用进行优化。特别是,传统SFM方法失败的挑战性场景的数据集可以用这种方法进行分解。 *  *  ### Neural Matching Fields: Implicit Representation of Matching Fields for Visual Correspondence * NeMF建议利用神经场进行语义对应匹配。一个粗略的成本量被用来指导一个高精度的神经匹配场。 *  *  *  ### SNAKE: Shape-aware Neural 3D Keypoint Field * SNAKE建议引入神经场的形状重建来帮助点云的三维关键点检测。不需要对检测进行监督。 *  *  *  ### 3D Concept Grounding on Neural Fields * NeRFs中的分割和概念学习可以从本文所提的方法中受益,每个空间位置的高维特征描述符都来自语言嵌入。用 NeRF 协助VQA。 *  *  *  ### ULNeF: Untangled Layered Neural Fields for Mix-and-Match Virtual Try-On * ULNef特别针对虚拟试穿环境,其中有多件服装被分层。这些层被建模为神经场,碰撞处理直接在场上进行。 * 只要优化目标函数,衣服就不会穿模。 *  *  *  ### Reinforcement Learning with Neural Radiance Fields * 在NeRF-RL中,神经场被用来学习一个潜伏空间,作为强化学习算法的状态表示。 *  *  ### PeRFception: Perception using Radiance Fields * 这是个新数据集,感觉可以做不少工作。 * PeRFception为感知相关的任务建立了新的大规模数据集,如分割、分类等。他们还在这些数据集上评估了一个plenoxels变体。 *  * 
上一篇:
Siggraph2022 NeRF 相关
下一篇:
ECCV2022 NeRF 相关
0
赞
207 人读过
新浪微博
微信
腾讯微博
QQ空间
人人网
提交评论
立即登录
, 发表评论.
没有帐号?
立即注册
0
条评论
More...
文档导航
没有帐号? 立即注册