wuvin
Always take risks!
Toggle navigation
wuvin
主页
实验室的搬砖生活
机器学习
公开的学术内容
公开的其他内容
About Me
归档
标签
友情链接
ZYQN
ihopenot
enigma_aw
hzwer
杨宗翰
CVPR2023 部分文章
2022-12-06 15:48:26
380
0
0
wuvin
# SinGRAF: Learning a 3D Generative Radiance Field for a Single Scene * 和我之前做 SinRGAN 的思路类似,但setting不一样。 * 给定一组图片(100张),训练生成模型生成多种符合条件的场景。 * 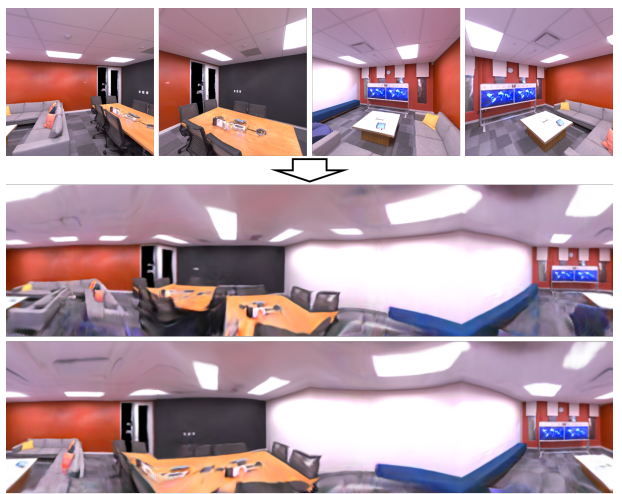 * 结构很简单,StyleGAN + tri-plane + patch discriminator。 * 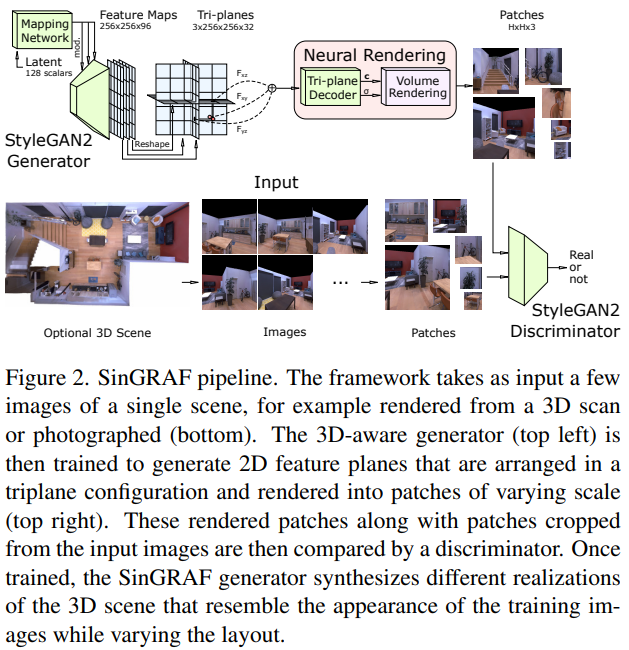 # RenderDiffusion: Image Diffusion for 3D Reconstruction, Inpainting and Generation * 去噪方式为把有噪音的图像先映射到三维空间,再渲染出来去噪。 * 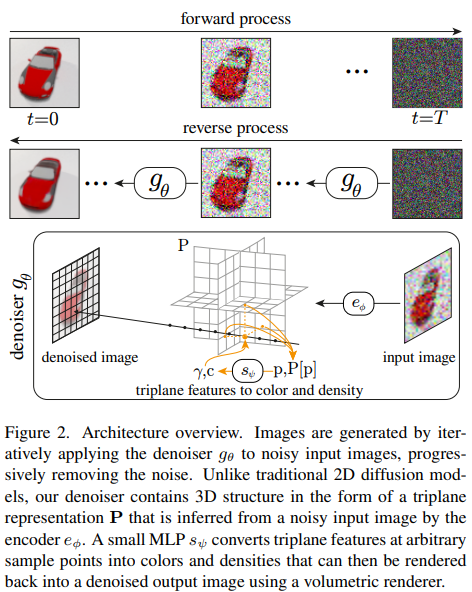 * 其中第一步为把 $M\times M\times 3$ 的2D图像通过一个Unet到 $N\times N\times 3\times n_f$ 的 triplane,然后调用 triplane 的可微渲染。 *  * 效果看起来持平 PixelNeRF,低于ED3D(基于Triplane的pi-gan) * 数值化指标只有重建 *  * **2D-3D直接用Unet也太粗糙了一点吧,或许可以试试把学好的表征的triplane用来diffuse** # DiffRF: Rendering-Guided 3D Radiance Field Diffusion * 纯 voxel 的 diffusion,且缺少量化指标。而且point-voxel diffusion 那篇已经把这个作为baseline干过了。 * 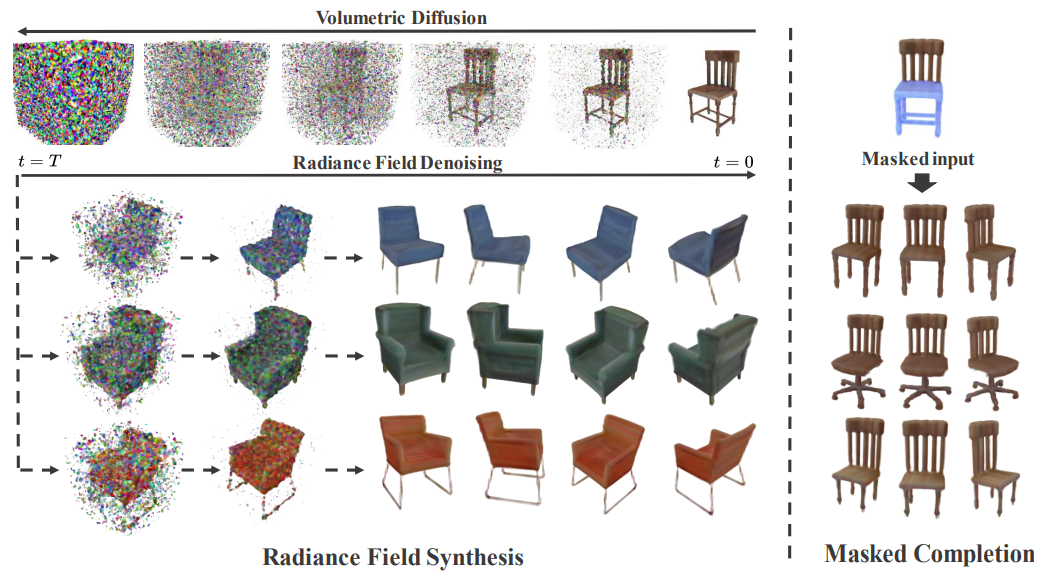 *  # LADIS: Language Disentanglement for 3D Shape Editing * 基于文字控制的三维编辑,但是效果不咋地,只在椅子上做了实验,我怀疑它中不了CVPR * 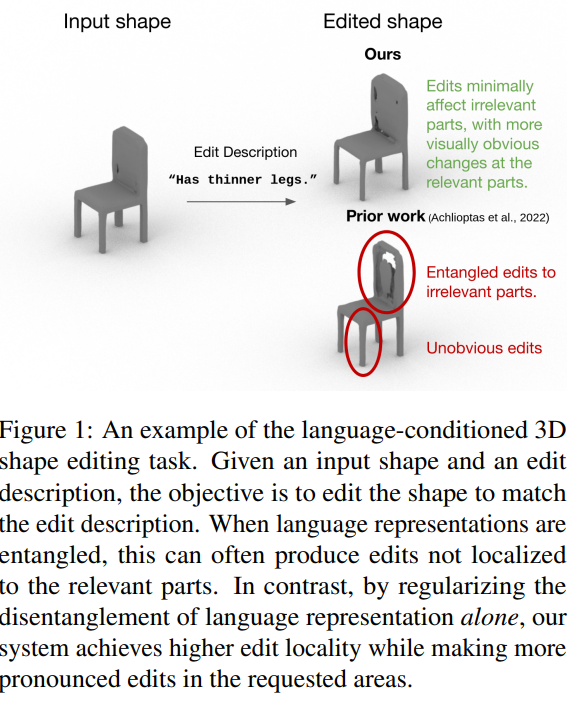 # Peekaboo: Text to Image Diffusion Models are Zero-Shot Segmentors * 用 Text to Image Diffusion Models 做分割 *  *  # 3DDesigner: Towards Photorealistic 3D Object Generation and Editing with Text-guided Diffusion Models *  * 一个看起来不明所以的架构 *  * 怎么连CLIP-NeRF 都打不过啊! *  * paper 里全只做了汽车数据集,看了下视频,三维一致性有点问题。
上一篇:
3D Diffusion
下一篇:
ICLR2023 论文选读
0
赞
380 人读过
新浪微博
微信
腾讯微博
QQ空间
人人网
提交评论
立即登录
, 发表评论.
没有帐号?
立即注册
0
条评论
More...
文档导航
没有帐号? 立即注册