wuvin
Always take risks!
Toggle navigation
wuvin
主页
实验室的搬砖生活
机器学习
公开的学术内容
公开的其他内容
About Me
归档
标签
友情链接
ZYQN
ihopenot
enigma_aw
hzwer
杨宗翰
近期2D GAN生成3D的论文
2023-07-02 20:50:52
123
0
0
wuvin
* 一些比较有意思的 cite 的 Score jacobian chaining 的工作。 # 3D-aware Image Generation using 2D Diffusion Models * 见到的第二篇深度估计+RGBD补全的文章了。 * 视频效果在https://jeffreyxiang.github.io/ivid/,厉害的是不仅做了forward facing的场景,还做了360度的,整体效果不错。 * 虽然说,这类靠深度估计+RGBD补全的,得到的效果会比较像石头的密度,但是 * 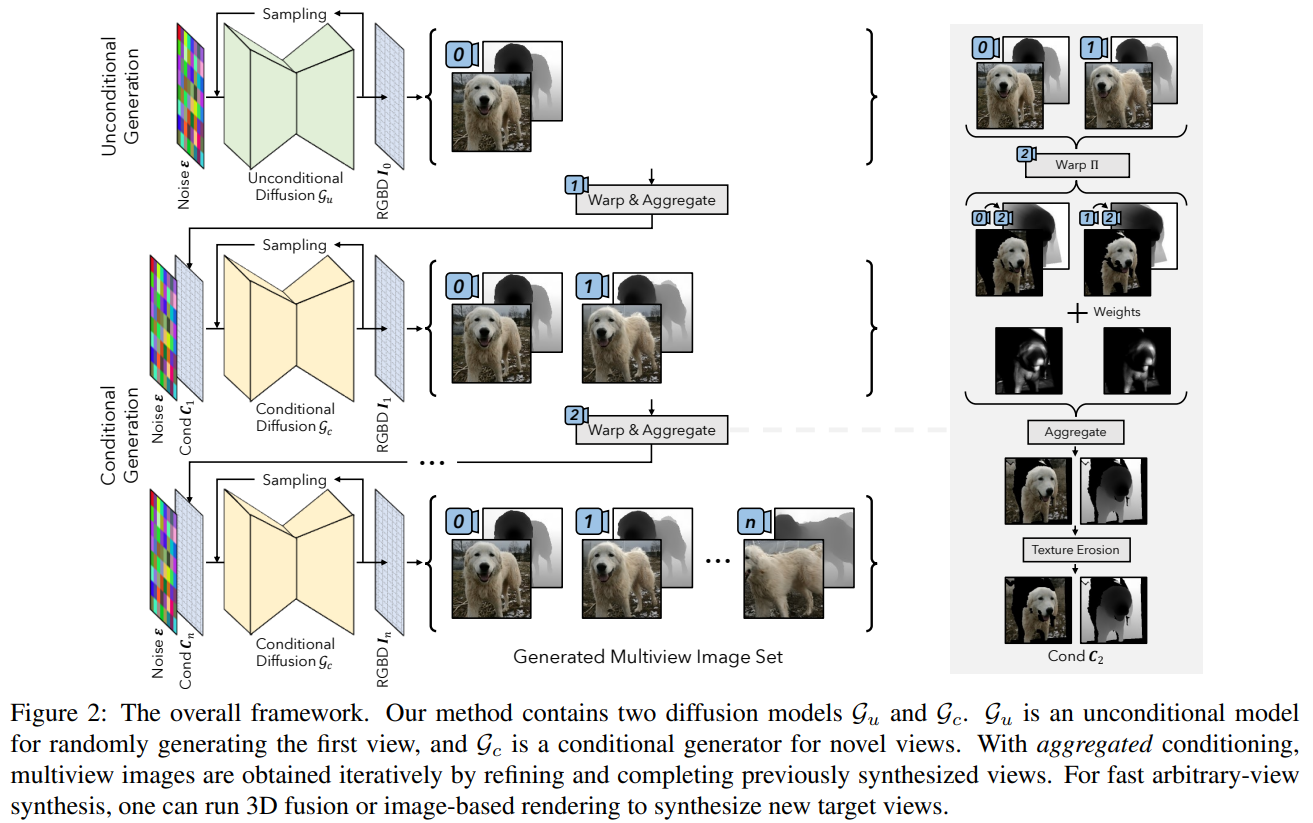 * warping 操作特地训练了生成模型 * 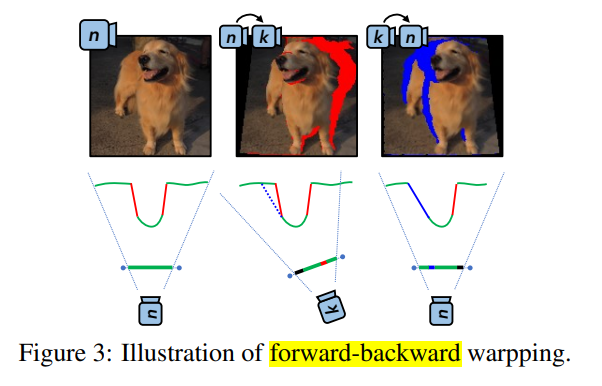 # TextDeformer: Geometry Manipulation using Text Guidance * 把SDS的思路用在了mesh deformation,不过本文引导使用的是 CLIP。 * 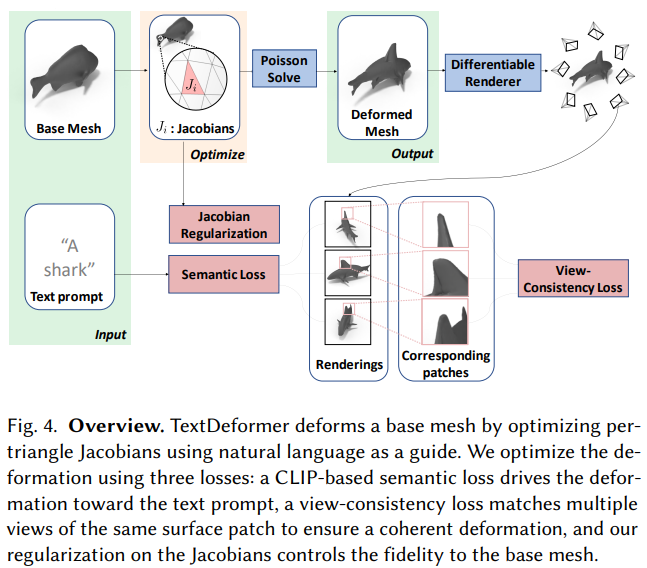 # Zero-1-to-3: Zero-shot One Image to 3D Object * 在合成数据上训练了一个去噪模型,具有良好的泛化能力,能够给出物体新视角高质量数据(虽然三维一致性一般)。 *  * 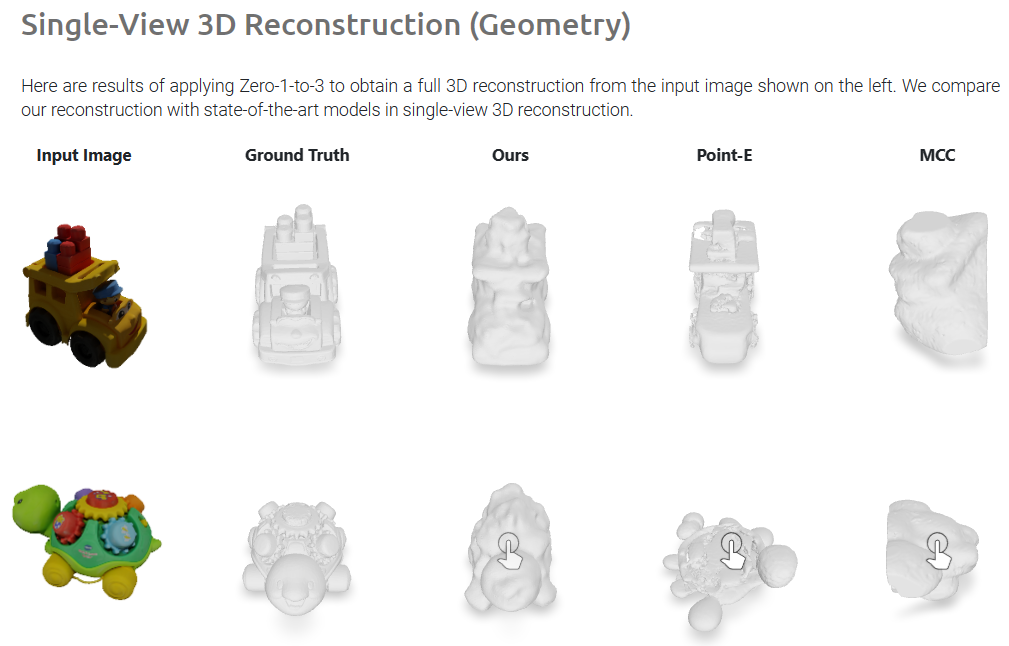 # Sin3DM: Learning a Diffusion Model from a Single 3D Textured Shape * 大概就是之前想做的单NeRF生成的改进版,基本思路一致——GAN换成Triplane Diffusion。 * 文章重新选择了更好的适用的数据集——普通数据难以应用、规则的材质化的效果才比较好。不再追求泛化能力,转而在局部场景上追求更好的质量。 *  * 相比之前的直接用3D版本SinGAN的效果: * 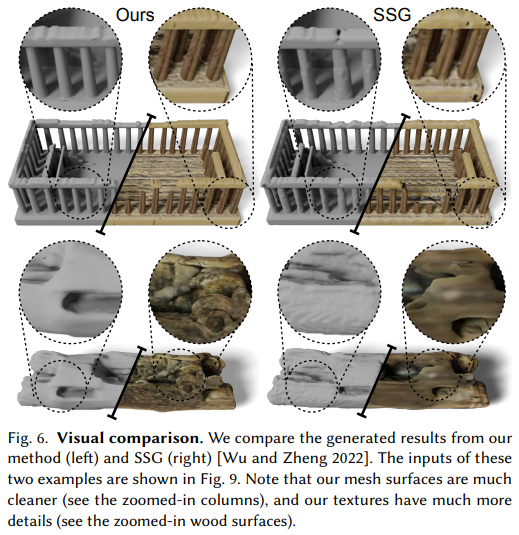 * 几何提升有限,但材质生成提升较好。 * **New Idea**: 结合 3D seam carving效果会怎么样呢?!3D seam carving规则化给出一个场景的不同尺寸,然后用于训练这个生成模型,从而能否提高模型对于场景应用的泛化能力? # ProlificDreamer: High-Fidelity and Diverse Text-to-3D Generation with Variational Score Distillation * **最近的少有的真正改进SDS质量的文章**,简称 VDS * 与SDS最大的区别在于认为要拟合的目标是一个分布,而不是一个三维场景样本。然后同时会优化多个样本(用多个样本来近似刻画分布,实际上也就四个),同时用这些样本 finetune一个LoRA,来表示目标物体的分布。 * 公式上的差异很小 *  *  * 公式里没有$\epsilon$是因为抵消掉了 *  * 因为推导时的优化目标是 *  * 其中$p_t$ 是指真实三维分布(即原版SD),而$q_t$则是根据当前场景渲染得到的物体的分布(也就是lora拟合的目标)。 * 以下是消融实验: *  * 可以看到每一步都提供了一个显著的质量提升。当然文章中还要很多其他细节(例如几何与纹理生成是分开的) *  *  *  * 
上一篇:
AIGC 新文章
下一篇:
NeRF 不确定性估计相关
0
赞
123 人读过
新浪微博
微信
腾讯微博
QQ空间
人人网
提交评论
立即登录
, 发表评论.
没有帐号?
立即注册
0
条评论
More...
文档导航
没有帐号? 立即注册