wuvin
Always take risks!
Toggle navigation
wuvin
主页
实验室的搬砖生活
机器学习
公开的学术内容
公开的其他内容
About Me
归档
标签
友情链接
ZYQN
ihopenot
enigma_aw
hzwer
杨宗翰
近期单视角三位生成论文
2023-11-20 13:10:00
113
0
0
wuvin
* 从 Zero123 开始数吧 # Zero-1-to-3: Zero-shot One Image to 3D Object * 通过在objaverse 上大规模训练得到具有一定泛化能力的,condition on 相机视角和参考图的 Diffusion。 * 但并没有像 SD 那样强大的泛化能力。 * 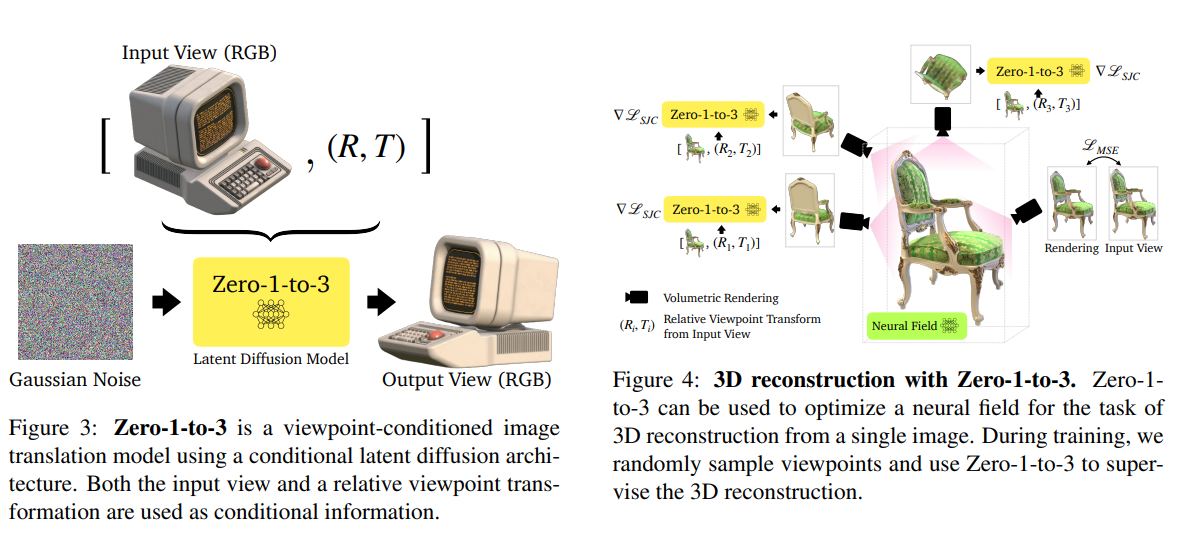 * 虽然有一些泛化能力,但后续文章指出,这泛化能力并不多 *  * 似乎相比同期方法有显著提升(但后续文章来看,SJC-I在这里被严重低估了): * 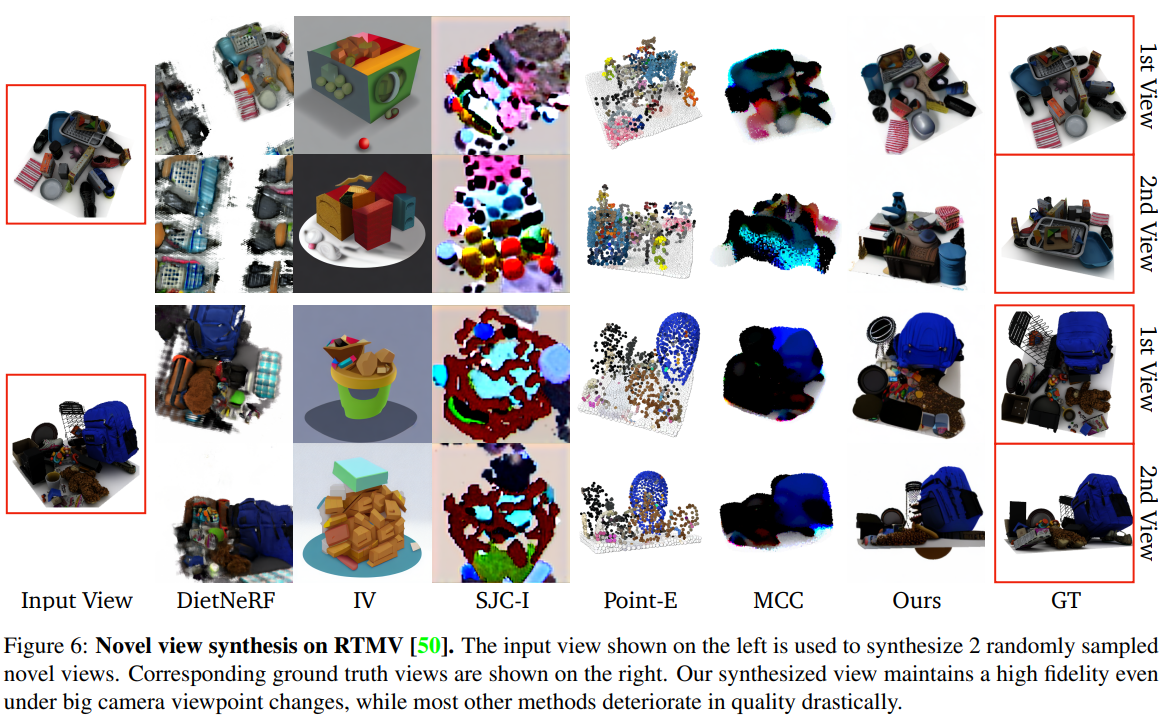 # Magic123: One Image to High-Quality 3D Object Generation Using Both 2D and 3D Diffusion Priors * 指出了 Zero-1-to-3 存在的问题—— OOD的情况下表现不好。 * 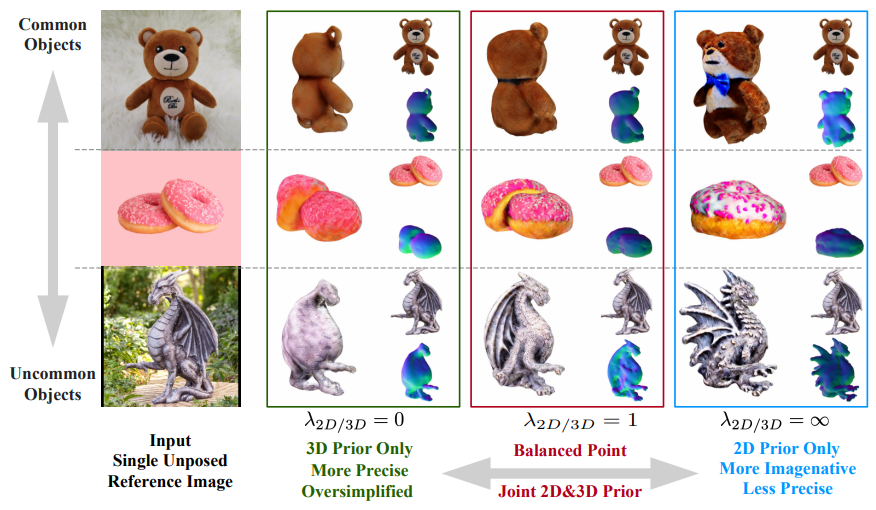 * 这里的2D prior 是指SDS,而3D prior 是指 Zero-1-to-3 * 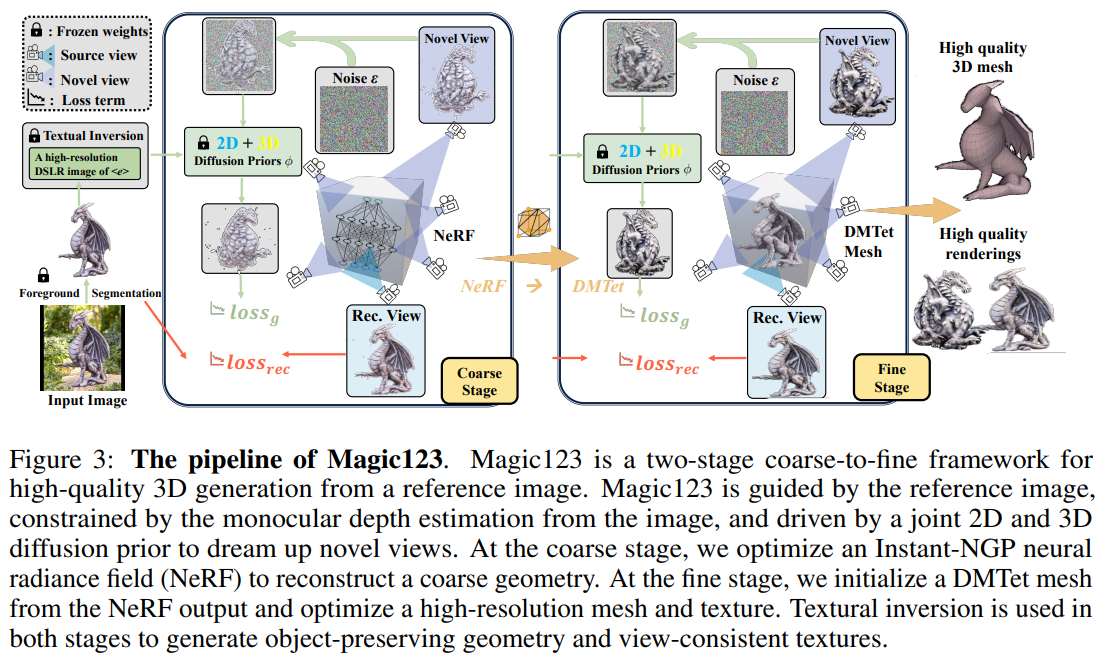 * 至少本文说明了,纯二维SDS只能做单视角生成的,没有Zero123说的那么差。 * 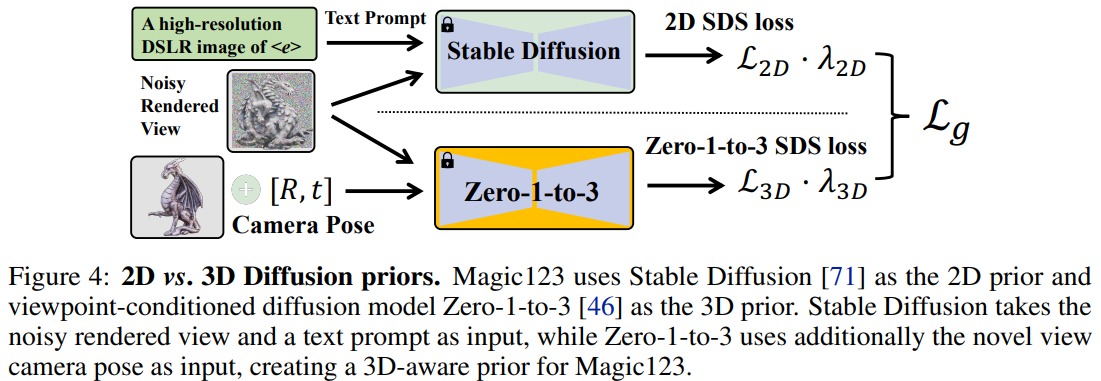 * 其实相比后续文章,这篇的数值指标是最差的(相对Zero-1-to-3没啥提升),但是确实唯一一个有泛化能力保障的。(即没有自己在中小规模数据上finetune) * 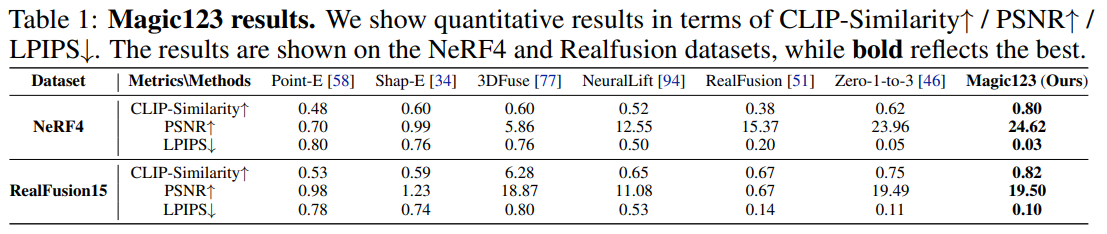 **接下来是有趣的三篇同期、同名工作** # Consistent-1-to-3: Consistent Image to 3D View Synthesis via Geometry-aware Diffusion Models * 这是三兄弟里最强的 * 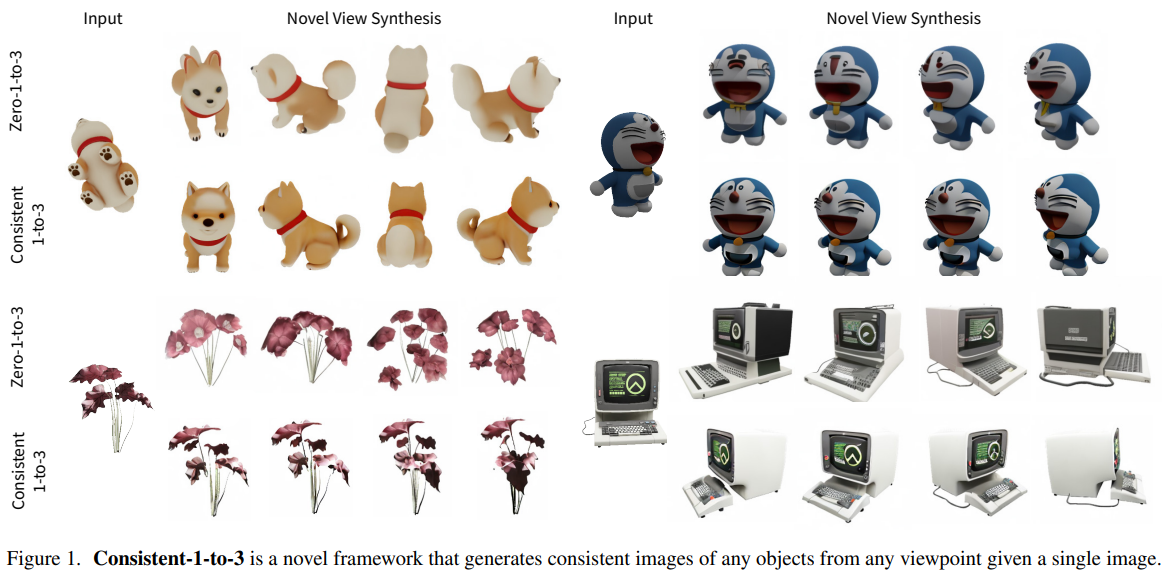 * 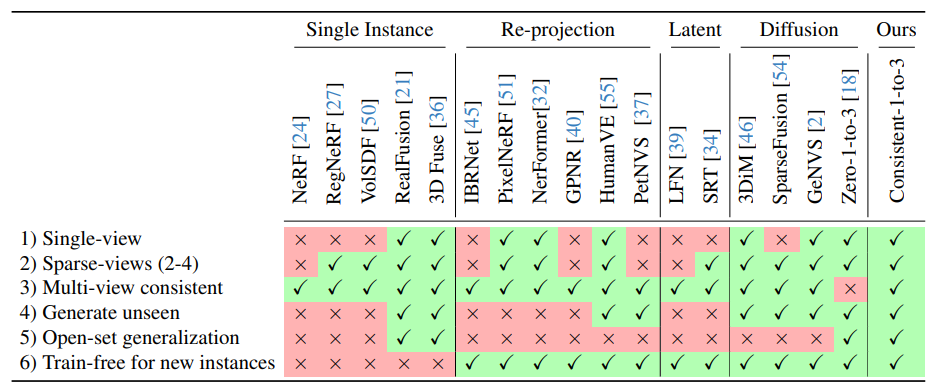 * UNet Attention 部分引入 epipolar 限制(epipolar在MVS等少视角重建真是屡试不爽) * 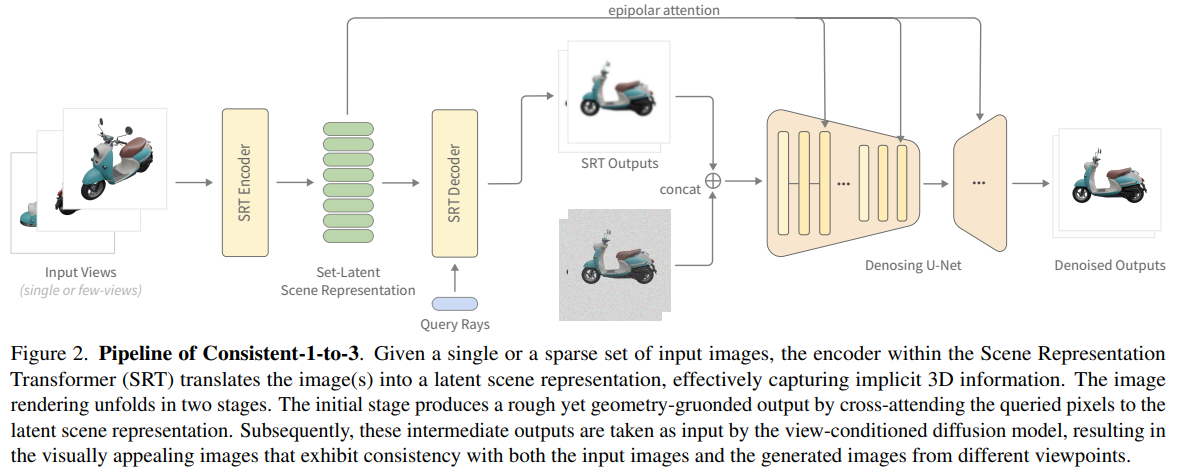 *  * 如同 Zero-123 一样,在Objaverse上训练的。需要注意的是,**和Zero123一样限制在单个物体,且必须白色背景!** # CONSISTENT123:ONE IMAGE TO HIGHLY CONSISTENT 3D ASSET USING CASE-AWARE DIFFUSION PRIORS * 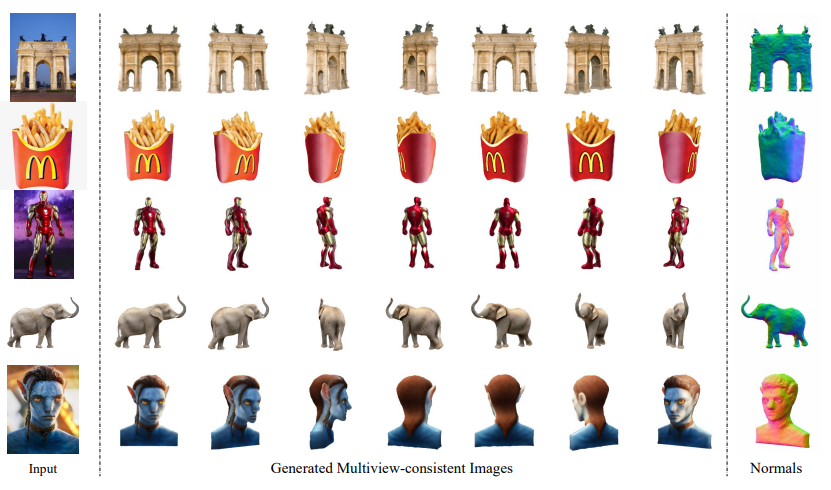 * 质量差一些,但是,没有训练新模型!保证了泛化能力。 *  * 感觉基于 Magic123的创新性少了些。 *  # CONSISTENT123: IMPROVE CONSISTENCY FOR ONE IMAGE TO 3D OBJECT SYNTHESIS *  * 思路更类似于 Zero123++,多个视角同时去噪,学习多视角联合分布。 *  # TOSS: HIGH-QUALITY TEXT-GUIDED NOVEL VIEW SYNTHESIS FROM A SINGLE IMAGE *  *  *  * 质量差一些,但是推理量更低的方案。(训练要8A100 7 天)。 # Wonder3D: Single Image to 3D using Cross-Domain Diffusion * 感觉质量更高的一篇 *  *  *  *  * Objaverse数据集,训练从 SD初始值开始,8卡A800 训练3天。 * 
上一篇:
ReadingList
下一篇:
近期2D-Diffusion生成3D的论文
0
赞
113 人读过
新浪微博
微信
腾讯微博
QQ空间
人人网
提交评论
立即登录
, 发表评论.
没有帐号?
立即注册
0
条评论
More...
文档导航
没有帐号? 立即注册